Predictive Analytics for Business Analysts
Predictive analytics is a vital component of modern business analytics, enabling organizations to forecast trends, mitigate risks, and identify opportunities. For business analysts, understanding predictive analytics is essential to delivering insights that drive decision-making. This article delves deep into predictive analytics, its applications, and how it empowers business analysts.
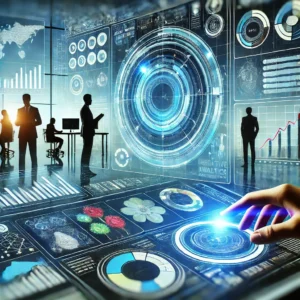
What is Predictive Analytics in Business Analytics?
Predictive analytics refers to the use of data, statistical algorithms, and machine learning techniques to identify the likelihood of future outcomes based on historical data. It bridges the gap between descriptive analytics (what has happened) and prescriptive analytics (what should be done).
In business analytics, predictive analytics is used to answer critical questions, such as:
- What will our sales look like next quarter?
- Which customers are likely to churn?
- How can we optimize our resources to maximize ROI?
For instance, an online retail company can use predictive analytics to forecast sales trends during holiday seasons, ensuring optimal stock levels and minimizing inventory costs.
What is a Real-Life Example of Predictive Analytics?
Predictive analytics is widely adopted across industries. Here’s an example from the healthcare sector:
Hospitals use predictive analytics to anticipate patient readmissions. By analyzing patient history, demographic data, and treatment outcomes, healthcare providers can identify high-risk patients and implement preventive measures.
Another example is fraud detection in banking. Banks leverage predictive models to detect unusual transaction patterns and flag potential fraudulent activities, saving millions in losses annually.
What are the Four Steps in Predictive Analytics?
Implementing predictive analytics typically involves these four key steps:
- Data Collection:
Collect relevant and high-quality historical data from diverse sources like CRM systems, databases, and online platforms. - Data Preprocessing:
- Clean the data to remove inconsistencies.
- Transform data into a usable format.
- Handle missing values and outliers.
- Model Building:
Develop predictive models using techniques like regression, decision trees, or neural networks. This step may involve training and validating models to ensure accuracy. - Model Deployment and Monitoring:
Deploy the predictive model to make real-time predictions and continuously monitor its performance. Adjust the model as new data becomes available.
What are the Techniques Used in Predictive Analytics?
Various techniques form the backbone of predictive analytics:
- Regression Analysis: Used for forecasting and determining relationships between variables.
- Classification Algorithms: Categorize data, such as identifying fraudulent transactions.
- Clustering: Groups similar data points for insights, like customer segmentation.
- Time Series Analysis: Analyzes data over time to predict future trends.
- Neural Networks: Mimics human brain functions to recognize patterns and make complex predictions.
Prescriptive Analytics vs. Predictive Analytics
While predictive analytics predicts what might happen, prescriptive analytics suggests actionable steps to influence the outcomes.
- Predictive Analytics Example: Forecasting a 20% drop in product sales.
- Prescriptive Analytics Example: Recommending targeted promotions to boost sales.
Predictive Analytics Models
Predictive models are mathematical frameworks designed to provide forecasts. Some common models include:
- Linear Regression: Analyzes relationships between dependent and independent variables.
- Random Forests: Combines multiple decision trees to improve accuracy.
- Time Series Analysis: Evaluates historical data trends over time.
- Logistic Regression: Used for binary outcomes, such as yes/no predictions.
Predictive Analytics Tools
Business analysts rely on various tools to perform predictive analytics effectively. Popular tools include:
- IBM SPSS Statistics: A robust tool for statistical analysis and predictive modeling.
- SAS Advanced Analytics: Widely used for data mining and predictive analytics.
- Microsoft Azure Machine Learning: A cloud-based platform for building predictive models.
- Python and R: Programming languages with powerful libraries like TensorFlow and Scikit-learn for machine learning.
Predictive Analytics Techniques
Predictive analytics employs various techniques to derive actionable insights:
- Data Mining: Extracts hidden patterns and trends from large datasets.
- Machine Learning: Automates the creation of analytical models.
- Text Analytics: Analyzes unstructured text data, such as customer reviews.
- Sentiment Analysis: Understands customer sentiment through data analysis.
Predictive Analytics Case Study Examples
Retail Industry:
A retail chain implemented predictive analytics to optimize its inventory. By analyzing sales data and external factors like weather and holidays, they reduced overstock and out-of-stock issues, saving 20% in operational costs.
Manufacturing:
A manufacturing company used predictive analytics to forecast machine failures. This enabled proactive maintenance, reducing downtime by 30%.
Predictive Analytics is Based on Which of the Following?
Predictive analytics primarily relies on:
- Historical data
- Statistical models
- Machine learning algorithms
- Human expertise for interpretation
Predictive Analytics Examples
Predictive analytics finds applications across sectors:
- E-commerce: Forecasting customer churn and personalizing recommendations.
- Healthcare: Predicting disease outbreaks and patient outcomes.
- Banking: Assessing credit risks and detecting fraud.
- Logistics: Optimizing delivery routes and reducing costs.
Conclusion
Predictive analytics is a game-changer for business analysts. It enables data-driven decision-making, ensuring businesses stay competitive in a fast-paced environment. By mastering predictive analytics models, tools, and techniques, business analysts can uncover insights that drive growth and innovation.
Related Articles :
External Resources:
- Explore IBM’s Predictive Analytics Overview
- Discover Top Predictive Analytics Tools on Gartner

Business Analyst , Functional Consultant, Provide Training on Business Analysis and SDLC Methodologies.
Good V I should certainly pronounce, impressed with your website. I had no trouble navigating through all the tabs as well as related info ended up being truly easy to do to access. I recently found what I hoped for before you know it in the least. Quite unusual. Is likely to appreciate it for those who add forums or anything, website theme . a tones way for your client to communicate. Nice task..